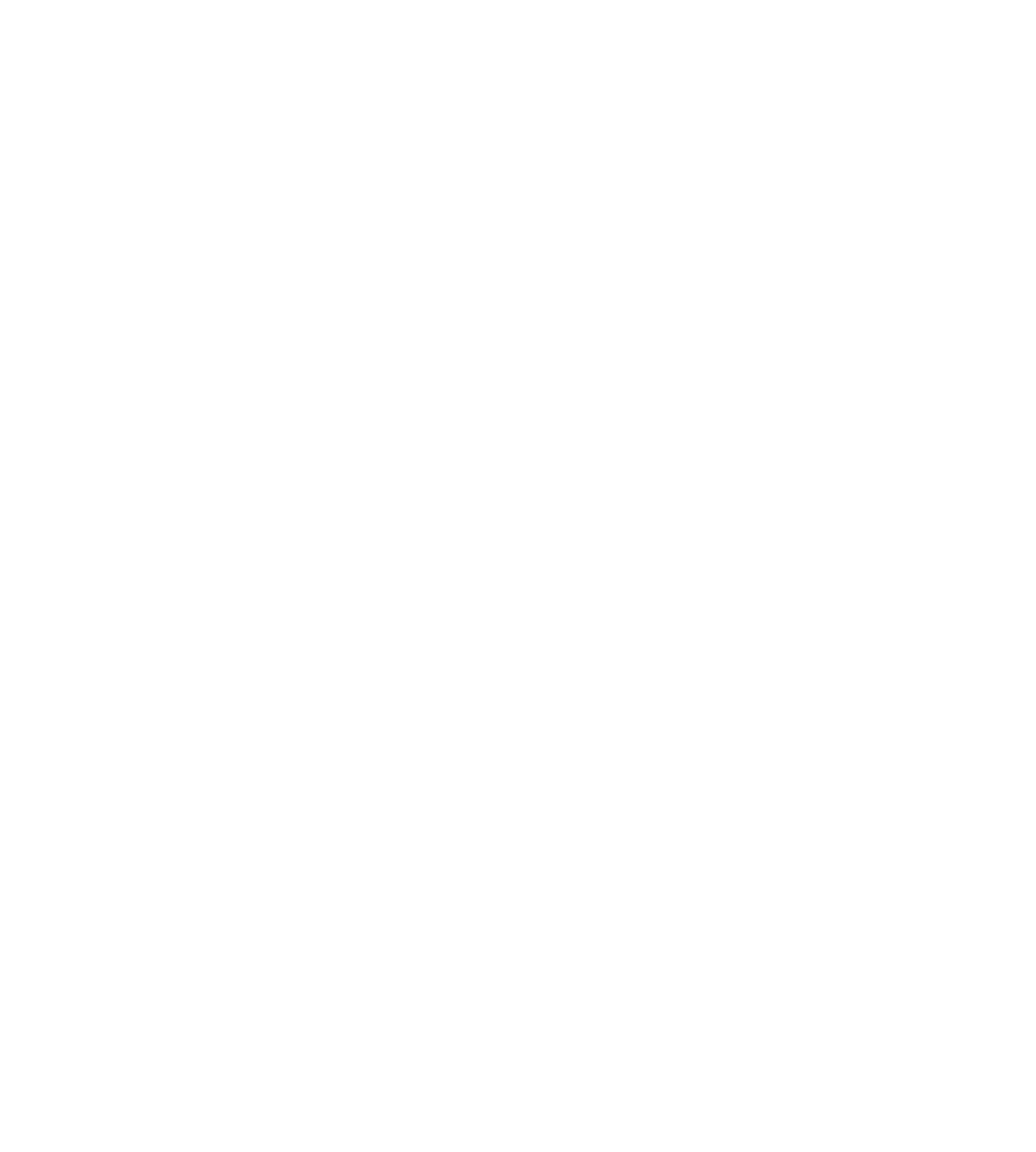
Stories from the University of Cambridge
CellphoneDB
-
Roser Vento-Tormo[1], Mirjana Efremova[1], Miquel Vento-Tormo[2], Sarah A. Teichmann[1],[3],[4]
1 Wellcome Sanger Institute, Cambridge, UK 2 YDEVS software development, Valencia, Spain 3 Theory of Condensed Matter Group, The Cavendish Laboratory, University of Cambridge, Cambridge, UK 4 European Molecular Biology Laboratory, European Bioinformatics Institute (EMBL-EBI), Cambridge, UK
-
2018
-
Sarah Teichman:
st9@sanger.ac.ukRoser Vento-Tormo:
rv4@sanger.ac.ukNicholas England:
ne6@sanger.ac.uk -
Vento-Tormo R, Efremova M, Botting RA et al. 2018. Single-cell reconstruction of the early maternal–fetal interface in humans. Nature 563, 347–353.
-
https://www.cellphonedb.org/index.html
-
European Molecular Biology Organization Long-Term Fellowship, European Research Council grants – ThDEFINE and ThSWITCH (EU), EU Future and Emerging Technologies -OPEN grant Grammar (EU), Human Frontier Science Program Long-Term Fellowship, Royal Society Dorothy Hodgkin Fellowship (UK), Wellcome Sanger core funding (UK), Wellcome Trust Investigator award (UK)
ABOUT THE OPEN-RESOURCE
Background
Understanding cell-to-cell communication mediated by ligand-receptor complexes is crucial for comprehending biological processes like development, differentiation, and inflammation. Investigating the crosstalk between different cell types provides insights into how physiological processes are facilitated. The scalability of single-cell genomics technologies has allowed for parallel analysis of multiple cells from the same tissue, providing a unique opportunity to study cell communication. However, existing tools for estimating ligand-receptor interactions faced two major issues: (i) the majority of ligands and receptors stored were not curated, leading to a lot of false positives (i.e. interactions stored in a database that do not exist in the reality); (ii) the existing methods did not account for the multimer nature of the majority of the ligand-receptor interactions, meaning each receptor (or ligand) consist by more than one subunit. The latter is important to make accurate predictions as it is the combination of subunits that gives specificity to the ligand/receptor interactions. To address these challenges, Dr Roser Vento-Tormo, group leader in the VenTo Lab, at Wellcome Sanger Institute, and collaborators developed CellphoneDB.
Function
CellphoneDB is a suite to study cell-to-cell communication from single-cell transcriptomics data. Identifying ligand–receptor interactions from single-cell RNA sequencing (scRNA-seq) requires both the annotation of complex ligand–receptor relationships from the literature (i.e. database) and a statistical method that integrates the resource with scRNA-seq data and selects relevant interactions from the dataset (ie. tool). CellphoneDB is composed of two units, a database and a tool. CellphoneDB database is a publicly available repository of curated receptors, ligands and their interactions. The database can be used to search for a particular ligand/receptor or in combination with the tool to interrogate your own single-cell transcriptomics data.
Development process
The development process was a highly collaborative and multidisciplinary work, that started in the Teichmann lab (additional information can be found here). “The computational approach was discussed multiple times in the lab meetings where multiple members from the Teichmann lab contributed to choose the best statistical approach used to infer ligand-receptor interactions.”, said Dr Vento-Tormo. The team also worked closely with experts in the field to ensure that receptor and ligand interactions stored in their database were correct. That included Gerry Graham, Professor at the University of Glasgow (additional information can be found here), an expert on cell-cell communication through chemokines. In addition, the group established collaborations with software developers that enabled to build the structure of the database, make the code scalable and have a webpage to query the ligand/receptor interactions present in the database.
One of the most challenging part in the development process was the scalability, explains Dr Vento-Tormo. “Since 2016, when we started the method, the amount of single cell generated has increased significantly and we had to account for this. Also, it was surprising how little we know about protein complexes (i.e. multimers) which are essential to understand receptors.”
Target user
In the first instance researchers. Also, anyone who wants to make their own synthetic sitting pathways, try some new combinations or expressing these new cell types, or using it for some new technology. CellphoneDB has a lot of translational potential and non-academic researchers are becoming more interested in it because the receptors are surface proteins that can be targeted with biologics.
Comparison to other technologies
The first differential of CellphoneDB database is that it only includes curated data, meaning that only interactions linked to a manuscript are included. Secondly, the database considers that the majority of receptors are multimers: in other words, that multiple subunits are required in order for the receptor to be functional. Thirdly, in later versions CellphoneDB also includes information about: a) non-protein ligands (e.g. steroid hormones), by taking into account the last enzyme involved in the production of the non-protein ligand; b) transcription factor and receptors that are part of the same signalling pathway. The link with downstream transcription factors, could be used as a sensor of the ligand-receptor interaction. Finally, the database and tool are continuously updated and made compatible with other tools to analyse single-cell genomics data.
IMPACT
Current use
On the GitHub CellphoneDB has been forked 103 times, and its publication has been cited more than 1300 times. Some examples of how to use it include: a) inferring function of a cell by quantifying the signals that is receiving or sending to other cells in the environment; b) comparing ligand/receptor interactions between two conditions (e.g. healthy vs disease); and c) informing specific in vitro protocol by knowing the signals that a specific cell is receiving from its surroundings (i.e. other cells).
Successful stories
The study published in Nature (2018) by Vento-Tormo and collaborators is one of the examples of the potential that CellphoneDB database has in inferring the communication between cells. The study resolves the maternal uterus-fetal placental communication during human pregnancy, and found that maternal uterine immune cells play a key role in placentation by downregulating inflammation (to avoid rejection) and promoting migration of the fetal placental cells into the uterus, an event crucial for pregnancy success. Another successful application of the database can be found in the publication by Garcia-Alonso and co-authors in Nature (2022), exploring the formation of follicle formation in the developing human ovaries. During development, the female eggs (carrying the genetic information) are surrounded by a specific cell type named “granulosa cells” forming the ovarian follicle. These structures are required for the future maturation of the egg. Using CellphoneDB, the authors could gain insights into the cell-cell communication events between the eggs and granulosa cells that are required to form these follicles.
Open source choice
“For us, it was essential for people to use CellphoneDB in their own research and for this, we had to opt for open source”, said Dr Vento-Tormo.
GOING FORWARD - WHERE TO IN THE NEXT 3-5 YEARS?
VenTo Lab would like their method to be integrated with other data modalities and statistical methods available. For this, they are continuously developing the tool and making it compatible with other formats required in other complementary single-cell genomics tools.
“For us, it was essential for people to use CellphoneDB in their own research and for this, we had to opt for open source.”
Roser Vento-Tormo
Overview of the database: (1) secreted and membrane proteins stored in protein_input; (2) protein complexes stored in complex_input; and (3) protein–protein interactions stored in interaction_input. © 2018, Wellcome Sanger Institute, licensed under CCC-BY-NC-ND 2.5 (https://creativecommons.org/licenses/by-nc-nd/2.5/). Reproduced from https://www.cellphonedb.org/index.html.
CellPhoneDB logo. © 2018, Wellcome Sanger Institute, licensed under CC-BY-NC-ND 2.5 (https://creativecommons.org/licenses/by-nc-nd/2.5/). Reproduced from https://www.cellphonedb.org/index.html.