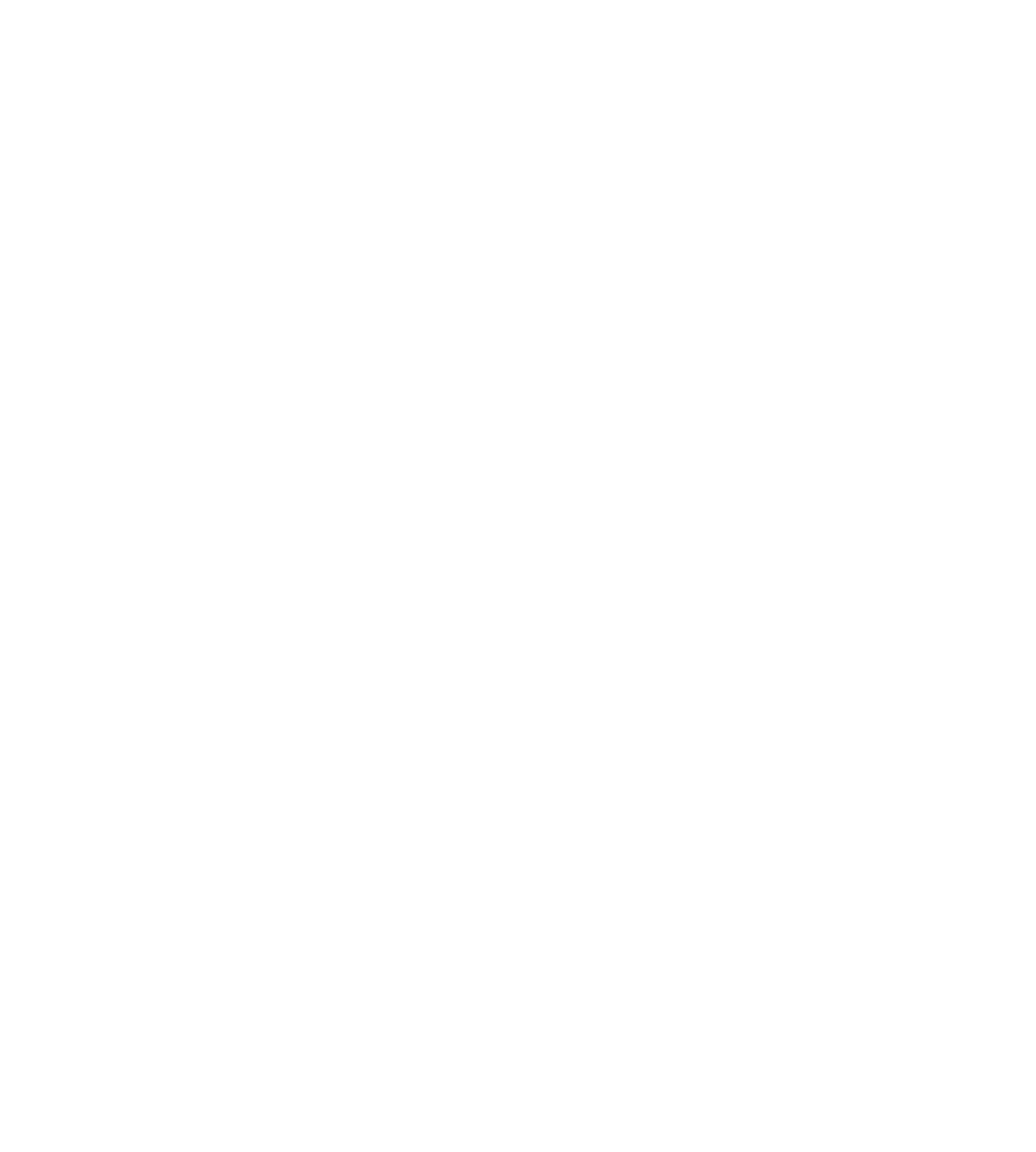
Stories from the University of Cambridge
SeedGerm
-
Joshua Colmer[1], Carmel M. O’Neill[2], Rachel Wells[2], Aaron Bostrom[1], Daniel Reynolds[1], Danny Websdale[1], Gagan Shiralagi[2], Wei Lu[3], Qiaojun Lou[4], Thomas Le Cornu[1], Joshua Ball[1], Jim Renema[5], Gema Flores Andaluz[5], Rene Benjamins[5], Steven Penfield[2], Ji Zhou[6],[7]
1 Engineering biology, Earlham Institute, Norwich Research Park, Norwich, UK 2 Crop Genetics, John Innes Centre, Norwich Research Park, Norwich, UK 3 College of Engineering, Nanjing Agricultural University, Nanjing, China 4 Shanghai Agrobiological Gene Center, Shanghai Academy of Agricultural Sciences, Shanghai, China 5 Syngenta Seeds BV, Enkhuizen, the Netherlands 6 State Key Laboratory of Crop Genetics and Germplasm Enhancement, Plant Phenomics Research Center, Jiangsu Collaborative Innovation Center for Modern Crop Production co-sponsored by Province and Ministry, Nanjing Agricultural University, Nanjing, China 7 Cambridge Crop Research, National Institute of Agricultural Botany, Cambridge, UK
-
2022
-
Colmer J, O’Neill CM, Wells R, Bostrom A, Reynolds D, Websdale D, Shiralagi G, Lu W, Lou Q, Le Cornu T, Ball J, Renema J, Andaluz GF, Benjamins R, Penfield S, Zhou J. 2020. SeedGerm: a cost-effective phenotyping platform for automated seed imaging and machine-learning based phenotypic analysis of crop seed germination. New Phytologist 228, 778-793.
-
https://github.com/Crop-Phenomics-Group/SeedGerm/releases
-
Biotechnology and Biological Sciences Research Council National Productivity Investment Fund Award, Norwich Research Park’s Biosciences Doctoral Training Partnership (UK), Jiangsu Collaborative Innovation Center for Modern Crop Production (China), NRP Translational Fund (UK), Syngenta Industrial Collaboration Fund (Switzerland), United Kingdom Research and Innovation Biotechnology and Biological Sciences Research Council Designing Future Wheat Strategic Programme (UK), United Kingdom Research and Innovation Biotechnology and Biological Sciences Research Council Core Strategic Programme in Resilient Crops (UK)
ABOUT THE OPEN-RESOURCE
Background
Effective seed germination and establishment are crucial characteristics for plant research and agricultural production. Routine germination scoring is still frequently based on visual inspection, but in large-scale experiments this task is time-consuming and prone to mistakes. Thinking about how to speed up and reduce errors in seed phenotyping methods, Prof Ji Zhou and collaborators developed the SeedGerm system, an imaging tool that could have wide ranging applications in both research and industrial contexts.
Function
SeedGerm is a platform designed for automating seed imaging and high throughput germination analysis, and it works in a variety of crop seeds. It combines cost-effective hardware and open source software for seed germination experiments, automated seed imaging, and machine-learning based phenotypic analysis. The software can process multiple image series simultaneously and produce reliable analysis of germination- and establishment-related traits, in both comma-separated values (CSV) and processed images (PNG) formats.
Development process
Prof Zhou explains that SeedGerm development was an iterative approach for hardware and software development. To make SeedGerm results reliable and reproducible, a lot of steps needed to be optimised during the development process. A software calibration has been added to the system to improve accuracy for low-quality images. Also, a number of tests using different sowing distances have been performed, and the results found that a distance of at least 1 cm between seeds improves the accuracy of the analysis. As expected when working with imaging processing, the task can be highly demanding on computing resources. Therefore, the developers do not recommend processing multiple image series simultaneously on an average computer.
Comparison to other technologies
SeedGerm is the first open scientific platform that combined imaging hardware (powered by Raspberry Pi, a computer and tailored control software) and automated trait analysis software (using a range of machine learning and computer vision techniques) to analyse germination-related traits that are key to seed science research and the assessment of seed vigour.
IMPACT
Current use
The system has been applied to a range of germination experiments for five important crop species: tomato, pepper, barley, and maize, and it was able to match seed specialists’ observations for the scoring of germination. Also, SeedGerm was successfully used to characterise morphological traits, such as seed size, width and length, providing insights into the physiological process by which seeds germinate. As far as the developers know, there are many academic and industrial research groups using the system, including John Innes Centre (UK), Nanjing Agricultural University (China), NIAB (UK),
GOING FORWARD - WHERE TO IN THE NEXT 3-5 YEARS?
As the morphology of different crop seeds vary greatly, it can be difficult to transfer some morphological measurements from one species to another. Therefore, the application of online learning or transfer learning tools may be advantageous for future developments of SeedGerm.
The pseudo colour indicated germination time, which enabled to assess seed germination related traits with temporal information. © 2023, Ji Zhou, licensed under CC-BY 4.0.
The application of online learning or transfer learning tools may be advantageous for future developments of SeedGerm.